Real-Time Artificial Intelligence (AI): Technologies, Applications, and Future Trends
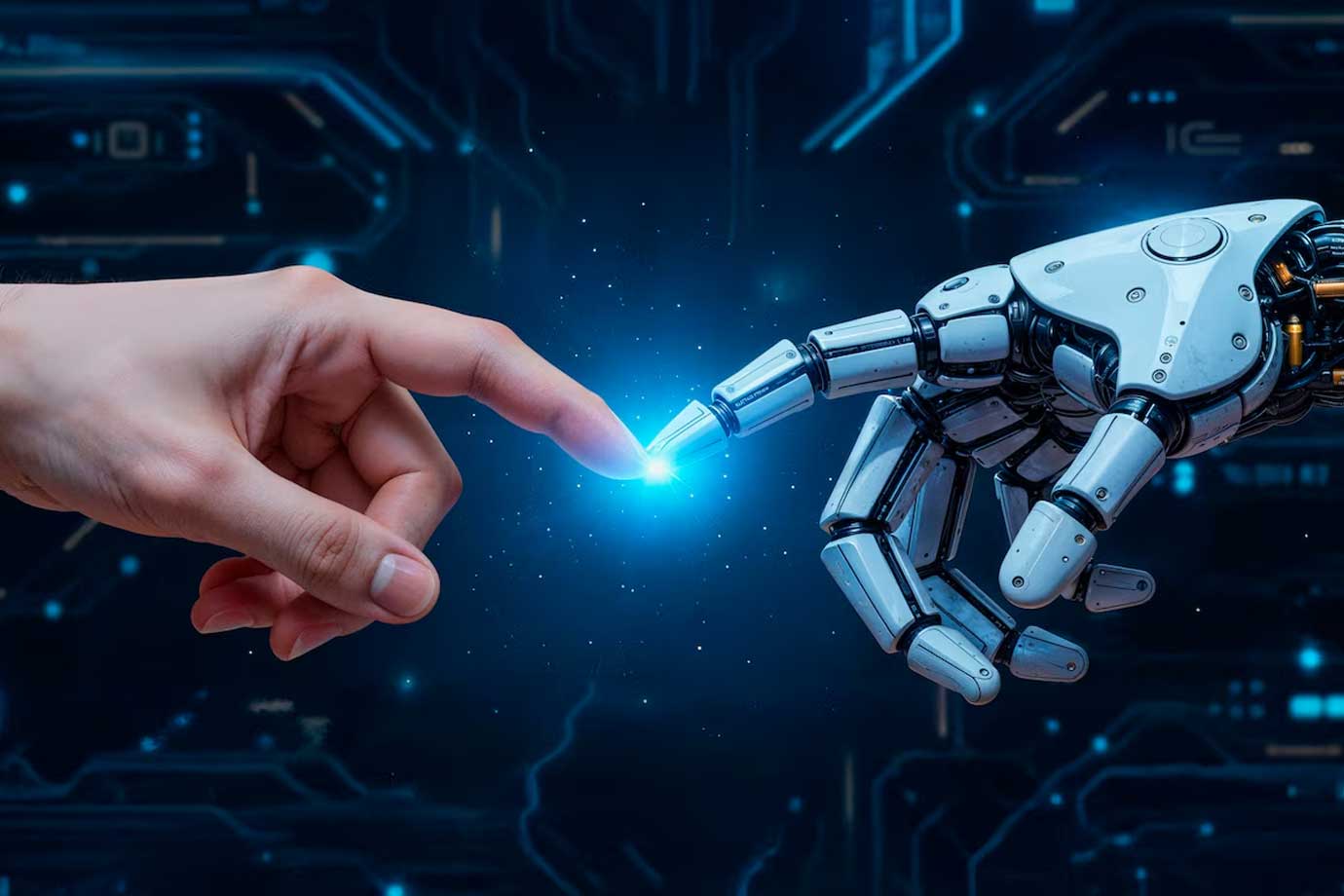
11 Apr 2025
Imagine a world in which computers not only compute information but also react at once, anticipating mishaps before they happen, detecting fraud, or interpreting languages in real time.
Real-time artificial intelligence (AI) can change industries and redefine what is believed to be possible when intelligence, speed, and response are blended together.
Whereas conventional AI takes a look back at historical data in the background, real-time artificial intelligence (AI) works now. It maximizes streaming data, allowing millisecond decisions and dynamic responses to be uninterrupted.
From trading platforms powered by AI and self-driving cars to tailored medicine, real-time artificial intelligence (AI) is making processes more efficient, accurate, and automated.
So how does the technology work? What drives its lightning-fast replies? And where will it head next?
In this blog, we’ll explore the cutting-edge technologies, applications, and future trends that are shaping the real-time artificial intelligence (AI) revolution.
I. Introduction
Artificial Intelligence (AI) has transformed businesses by enabling machines to examine data, find patterns, and make decisions. Earlier, AI systems operated in batch mode and gathered, processed, and analyzed data at fixed intervals.
Nevertheless, live AI systems with the capability of instant or nearly instant data processing and generation of insight exist due to the necessity of making speedier response decisions.
Real-time artificial intelligence (AI) is essential to modern applications, from healthcare networks that spot medical anomalies as they occur to autonomous vehicles that make split-second decisions.
The shift to real-time artificial intelligence (AI) from classical AI with chatbots offering real-time, human-like meetings and AI-powered trading algorithms responding in milliseconds, artificial intelligence (AI) in finance has completely changed user experience, safety, and efficiency across a range of industries.
II. Core Technologies Enabling Real-time Artificial Intelligence (AI)
1. Edge Computing
The edge computing technique is highly suitable for IoT devices, smart cameras, and self-driving cars, in which high latency could result in major disasters. In this scenario, edge computing reduces latency to assist in delivering real-time decision-making.
2. Streaming Data Processing
The streaming data processing systems Apache Kafka, Apache Flink, and AWS Kinesis control continuous data flow for real-time artificial intelligence, which is essential for fraud detection, real-time monitoring, and predictive analytics in sectors including banking, healthcare, and cybersecurity.
3. Lightweight Machine Learning Models
Dropping unnecessary parameters and reducing numerical precision strategies optimize AI models for real-time efficiency. Wearables, smart home devices, and industrial sensors are low-power, resource-limited devices that can be used to apply AI using such frameworks as TensorFlow Lite and TinyML, supporting faster inference.
4. Hardware Acceleration
Hardware accelerators like GPUs, TPUs, and AI chips like NVIDIA Jetson and Google Coral provide high-performance computing in real-time use cases like robotics, video analytics, and trading systems with drastically sped-up AI computation.
III. Applications of Real-time Artificial Intelligence (AI)
-
Autonomous Systems
Self-driving cars: AI processes sensor data in real time to make driving decisions, avoid obstacles, and enhance safety. Drones: Autonomous drones use AI for navigation, object detection, and surveillance in industries like agriculture, defense, and logistics. Robotics: AI-powered robots in manufacturing, warehouses, and homes perform real-time decision-making for efficiency and automation. -
Healthcare
Real-time patient monitoring: Wearable devices and hospital sensors track vitals like heart rate and oxygen levels, alerting doctors to anomalies. AI-assisted surgeries: Robotic surgery systems use AI for precision, minimizing risks and improving outcomes. Wearable health tech: Smartwatches and fitness trackers provide real-time health insights, detecting irregularities like atrial fibrillation. -
Finance
Fraud detection: AI analyzes transaction patterns in real time to detect and prevent fraudulent activities. Algorithmic trading: AI-powered trading bots process market data instantly, executing high-speed trades for better financial outcomes. Risk assessment: Real-time artificial intelligence (AI) evaluates creditworthiness and market risks, helping financial institutions make informed decisions. -
Smart Cities
Traffic management: AI optimizes traffic signals, predicts congestion, and guides autonomous vehicles for smoother urban mobility. Energy grid optimization: Smart grids use AI to balance electricity demand and supply, reducing waste and improving efficiency. Emergency response: AI-driven surveillance and predictive analytics assist in disaster management, law enforcement, and first responder coordination. -
Consumer Tech
Voice assistants: AI-powered assistants like Alexa, Siri, and Google Assistant process speech in real time for seamless user interaction. Real-time translation: AI-driven translation tools enable instant multilingual communication for travelers and businesses. AR/VR: Augmented and virtual reality applications use real-time artificial intelligence (AI) for immersive gaming, virtual meetings, and interactive experiences.
IV. Challenges in Real-time Artificial Intelligence (AI) Development
1. Technical Challenges
-
Latency and Resource Constraints:
Real-time AI requires ultra-high-speed data processing, but there are network latency, computational, and infrastructure bottlenecks that can impede the process. -
Speed vs. Accuracy:
More accuracy often means complex models, and complex models are slow. AI needs to be made precise as well as efficient, which is a big problem. -
Processing Noisy or Incomplete Data Streams:
Real-time AI systems need to handle infinite data, which may be incomplete, inconsistent, or noisy. Effective filtering and error-handling techniques are required to make the system reliable.
2. Ethical and Regulatory Concerns
-
Problems with continuous data collection:
Real-time AI raises ethical and regulatory issues regarding privacy, consent, and data protection due to its continuous data collection practice. -
Real-time decision-making bias:
Biases in training data can be introduced into AI systems, and this can result in discriminatory or negative real-time decisions in places like banking, law enforcement, and employment. -
Compliance regulations (e.g., GDPR, HIPAA):
Organizations must ensure that real-time artificial intelligence adheres to statutory requirements for the protection of data, healthcare privacy, and consumer protection.
3. Scalability and Reliability
-
Ensuring systems perform under high load:
Real-time artificial intelligence (AI) applications in industries like finance and smart cities must handle massive data streams without performance degradation. -
Fail-safe mechanisms for critical applications:
Failure of AI has significant implications in high-risk contexts (e.g., autonomous vehicles and healthcare). To avoid disastrous failure, it is imperative to construct redundant, fault-tolerant systems.
V. Future Trends and Innovations
The development of real-time artificial intelligence (AI) is driven by various emerging technologies that enhance efficiency and scalability.
-
AI at the Edge
Decentralized AI designs ensure on-device real-time processing while minimizing cloud computing dependency. The change encourages latency, security, and efficiency for contingent applications across autonomous systems, industrial automation, and healthcare monitoring. -
5G and Real-Time AI Synergy
The combination of 5G networks and AI achieves ultra-low latency communication, triggering new applications, including autonomous vehicle coordination with remote-controlled robotic surgery and immersive AR/VR. High bandwidth is used to enable real-time AI applications to run seamlessly in uncertain environments. -
Neuromorphic Computing
Inspired by the human brain, Neuromorphic chips (e.g., Intel Loihi, IBM TrueNorth) are energy-friendly for real-time AI processing. The chips' learning adaptability and pattern recognition render them useful for robotics, smart wearables, and sensors. -
Ethical AI Frameworks
As real-time AI is fast maturing into an operational space, ethical issues of unfairness, transparency, and accountability have led to the development of bias detection models, explainable AI (XAI), and compliance standards such as GDPR and HIPAA. These regulations ensure the use of responsible and trustworthy AI. With these improvements, real-time AI is made faster, smarter, and more responsible for changing industries and affecting day-to-day life.
VI. Case Study: Real-time Artificial Intelligence (AI) in Action
Transforming Emergency Response with AI
Real-time artificial intelligence (AI) is revolutionizing emergency response by making it possible to make rapid, intelligent decisions when disaster strikes. AI-driven sensors, drones, and predictive models ready first responders to rapidly estimate the situation at hand to minimize response times and enhance the possibilities of successful rescue missions.
How Does Real-time Artificial Intelligence (AI) Function in Disaster Management?
-
Sensors and IoT Devices:
IoT devices and sensors would detect unusual activities such as earthquakes, fires, or infrastructure collapse, and emergency services would receive alerts in real time. -
AI drones:
AI drones would offer live airborne video to assist rescuers in finding survivors and assessing damage more rapidly. -
Predictive Models:
Predictive models analyze determinants of risk, including weather conditions, seismic activity, and historical disaster information, to optimally allocate resources.
Important Results and Takeaways
-
Shorter Response Times:
AI-driven insights enable quick emergency service deployment. -
Enhanced Situational Awareness:
AI minimizes human error in determining high-risk areas and analyzing big data. -
Scalability concerns:
Combining AI with existing emergency services requires heavy financial and infrastructure investment. Despite such challenges, real-time artificial intelligence (AI) continues to strengthen emergency response and make disaster management more proactive, efficient, and life-saving.
Conclusion
In conclusion, real-time artificial intelligence (AI) is reshaping industries such as emergency services, autonomous systems, medicine, and finance by dramatically enhancing efficiency and security. It drives significant advancements in edge computing, stream data processing, and compact machine learning models, ensuring faster and more cost-effective insights that are essential for success in today's data-driven landscape.
Yet, in optimal deployment, scaling requirements, ethics, and latency boundaries have to be solved. The world will become smart, safe, and connected because of the continuous development of 5G, neuromorphic computing, and ethical AI platforms.
We at Nanobyte Technologies offer the most excellent Real-Time Artificial Intelligence (AI) solutions that enable companies to avail the newest technology for driving efficiency and innovation.